RESEARCH
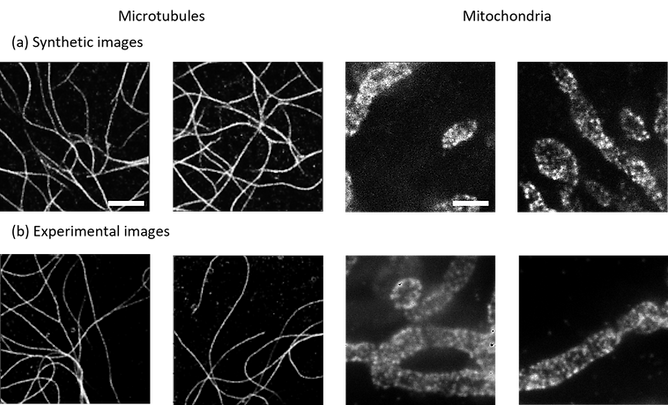
a) Example synthetic images of microtubules (alpha-tubulin – Alexa647) and mitochondria (TOM 22 – Alexa647) generated by our diffusion model. b) Example experimental super-resolution images, used as training data. Scale bars = 2.5 μm
This Microtubule Does Not Exist: Super-Resolution Microscopy Image Generation by a Diffusion Model
Generative models, such as diffusion models, enable the creation of high-quality data across various fields. In this work, we train a diffusion model on super-resolution microscopy images, showing that the generated images resemble experimental ones without memorization. To demonstrate the value of such synthetic images, we train a deep learning model for single-image super-resolution (SISR) on generated high-resolution data and compare its performance to a model trained on experimental or mathematically modeled images. We show that our approach improves the reconstruction quality and spatial resolution of the images. Additionally, our pipeline is user-friendly, publicly available online, and encourages future applications in the field.
A. Saguy, T. Nahimov, M. Lehrman, E. G. Mariscal, I. H. Cenalmor, O. Alalouf, A. Balakrishnan, M. Heilemann, R. Henriques, Y. Shechtman. "This Microtubule Does Not Exist: Super-Resolution Microscopy Image Generation by a Diffusion Model". Small Methods 2400672 (2024).
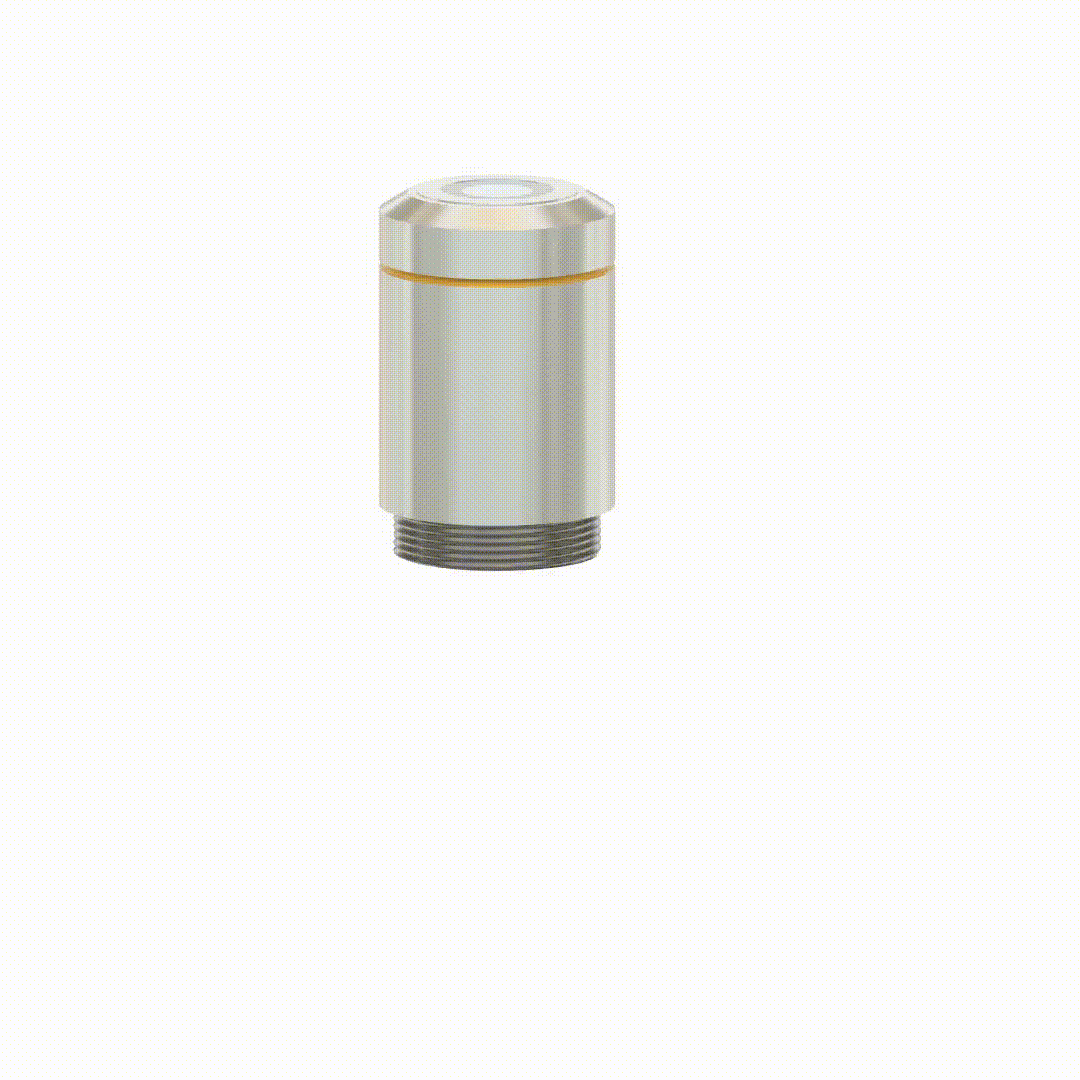
Depth-enhanced high-throughput microscopy by compact PSF engineering
High-throughput microscopy is vital for screening applications, where three-dimensional (3D) cellular models play a key role. However, due to defocus susceptibility, current 3D high-throughput microscopes require axial scanning, which lowers throughput and increases photobleaching and photodamage. Point spread function (PSF) engineering is an optical method that enables various 3D imaging capabilities, yet it has not been implemented in high-throughput microscopy due to the cumbersome optical extension it typically requires. Here we demonstrate compact PSF engineering in the objective lens, which allows us to enhance the imaging depth of field and, combined with deep learning, recover 3D information using single snapshots. Beyond the applications shown here, this work showcases the usefulness of high-throughput microscopy in obtaining training data for deep learning-based algorithms, applicable to a variety of microscopy modalities.
N. Opatovski, E. Nehme, N. Zoref, I. Barzilai, R. O. Kedem, B. Ferdman, P. Keselman, O. Alalouf, Y. Shechtman. "Depth-enhanced high-throughput microscopy by compact PSF engineering". Nature Communications 15, 4861 (2024).
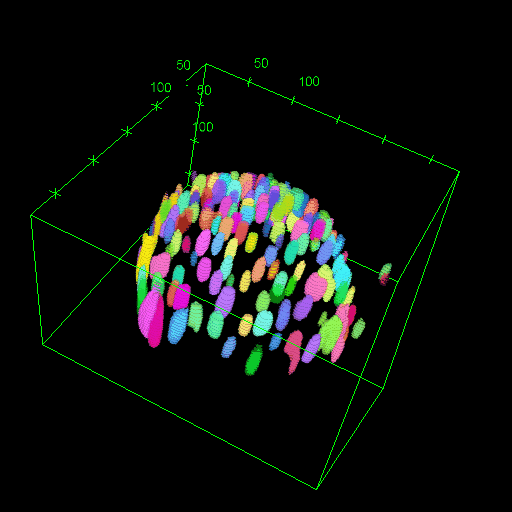
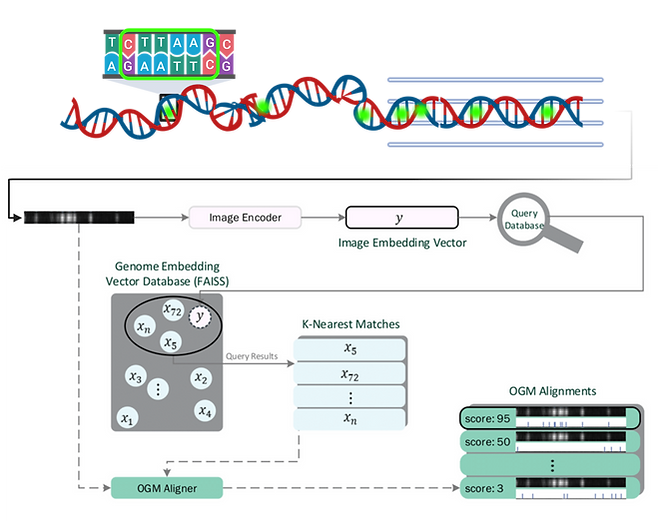
OM2Seq: Learning retrieval embeddings for optical genome mapping
Genomics-based diagnostic methods that are quick, precise, and economical are essential for the advancement of precision medicine, with applications spanning the diagnosis of infectious diseases, cancer, and rare diseases. One technology that holds potential in this field is optical genome mapping (OGM), which is capable of detecting structural variations, epigenomic profiling, and microbial species identification. It is based on imaging of linearized DNA molecules that are stained with fluorescent labels, that are then aligned to a reference genome. However, the computational methods currently available for OGM fall short in terms of accuracy and computational speed. This work introduces OM2Seq, a new approach for the rapid and accurate mapping of DNA fragment images to a reference genome. Based on a Transformer-encoder architecture, OM2Seq is trained on acquired OGM data to efficiently encode DNA fragment images and reference genome segments to a common embedding space, which can be indexed and efficiently queried using a vector database. We show that OM2Seq significantly outperforms the baseline methods in both computational speed (by two orders of magnitude) and accuracy.
Y. Nogin, D. Sapir, T. D. Zur, N. Weinberger, Y. Belinkov, Y. Ebenstein, Y. Shechtman. "OM2seq: Learning retrieval embeddings for optical genome mapping". Bioinformatics Advances (2024)
Large-FOV 3D localization microscopy by spatially variant point spread function generation
Accurate characterization of the microscopic point spread function (PSF) is crucial for achieving high-performance localization microscopy (LM). Traditionally, LM assumes a spatially invariant PSF to simplify the modeling of the imaging system. However, for large fields of view (FOV) imaging, it becomes important to account for the spatially variant nature of the PSF. Here, we propose an accurate and fast principal components analysis–based field-dependent 3D PSF generator (PPG3D) and localizer for LM. Through simulations and experimental three-dimensional (3D) single-molecule localization microscopy (SMLM), we demonstrate the effectiveness of PPG3D, enabling super-resolution imaging of mitochondria and microtubules with high fidelity over a large FOV. A comparison of PPG3D with a shift-variant PSF generator for 3D LM reveals a threefold improvement in accuracy. Moreover, PPG3D is approximately 100 times faster than existing PSF generators, when used in image plane–based interpolation mode. Given its user-friendliness, we believe that PPG3D holds great potential for widespread application in SMLM and other imaging modalities.
D. Xiao, R. K. Orange, N. Optaovski, A. Parizat. E. Nehme, O. Alalouf, Y. Shechtman. "Large-FOV 3D localization microscopy by spatially variant point spread function generation". Science Advances 10, eadj3656 (2024)
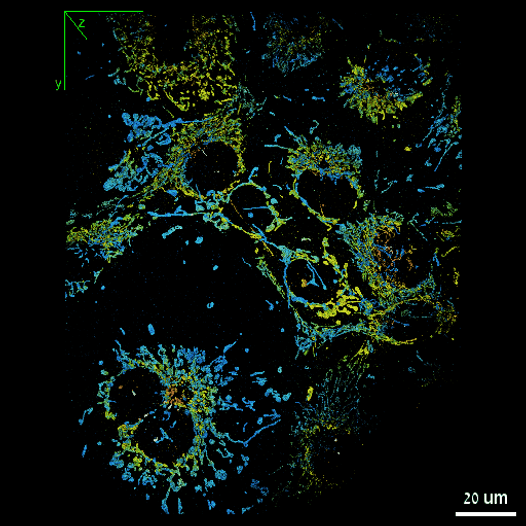
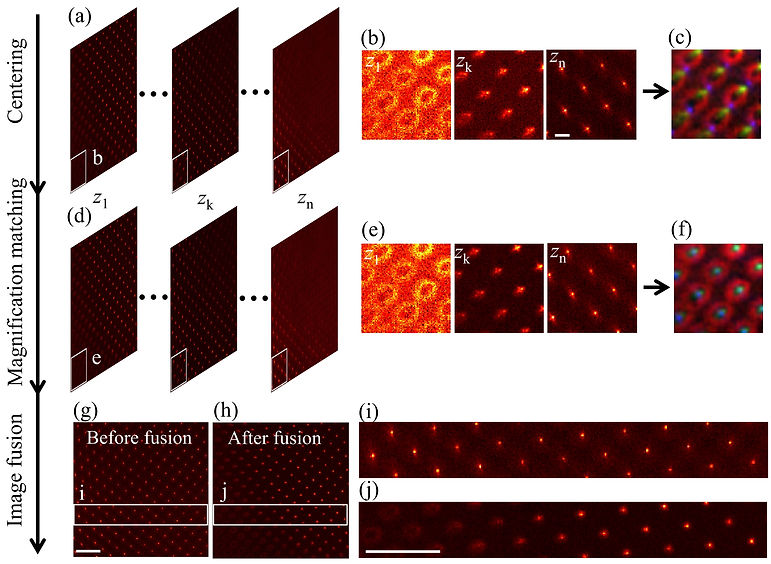
All-in-focus large-FOV GRIN lens imaging by multi-focus image fusion
Gradient refractive index (GRIN) lenses are useful for miniaturized and in-vivo imaging. However, the intrinsic field-dependent aberrations of these lenses can deteriorate imaging resolution and limit the effective field of view. To address these aberrations, adaptive optics (AO) has been applied which inevitably requires the incorporation of additional hardware. Here we focus on field curvature aberration and propose a computational correction scheme which fuses a z-stack of images into a single in-focus image over the entire field of view (FOV). We validate our method by all-in-focus wide-field imaging of a printed letter sample and fluorescently labeled mouse brain slices. The method can also provide a new and valuable option for imaging enhancement in the scanning-modality use of GRIN lens microscopy.
D. Xiao, Z. Lin, Y. Shechtman. "All-in-focus large-FOV GRIN lens imaging by multi-focus image fusion". Optics Continuum 2, 11, 2290-2297 (2023)
Near index matching enables solid diffractive optical element fabrication via additive manufacturing
Diffractive optical elements (DOEs) have a wide range of applications in optics and photonics, thanks to their capability to perform complex wavefront shaping in a compact form. However, widespread applicability of DOEs is still limited, because existing fabrication methods are cumbersome and expensive. Here, we present a simple and cost-effective fabrication approach for solid, high-performance DOEs. The method is based on conjugating two nearly refractive index-matched solidifiable transparent materials. The index matching allows for extreme scaling up of the elements in the axial dimension, which enables simple fabrication of a template using commercially available 3D printing at tens-of-micrometer resolution. We demonstrated the approach by fabricating and using DOEs serving as microlens arrays, vortex plates, including for highly sensitive applications such as vector beam generation and super-resolution microscopy using MINSTED, and phase-masks for three-dimensional single-molecule localization microscopy. Beyond the advantage of making DOEs widely accessible by drastically simplifying their production, the method also overcomes difficulties faced by existing methods in fabricating highly complex elements, such as high-order vortex plates, and spectrum-encoding phase masks for microscopy.
R. O. Kedem, N. Opatovski, D. Xiao, B. Ferdman, O. Alalouf, S. K. Pal, Z. Wang, H. Emde, M. Weber, S. J. Sahl, A. Arie, S. W. Hell, Y. Shectman. "Near index matching enables solid diffractive optical element fabrication via additive manufacturing". Light Science & Applications 12, 222 (2023)
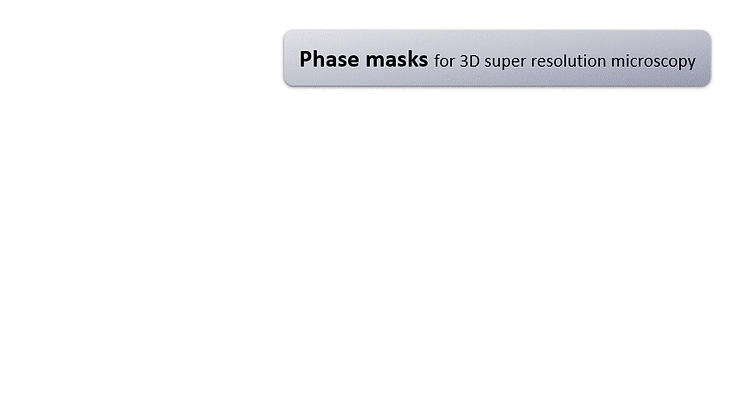
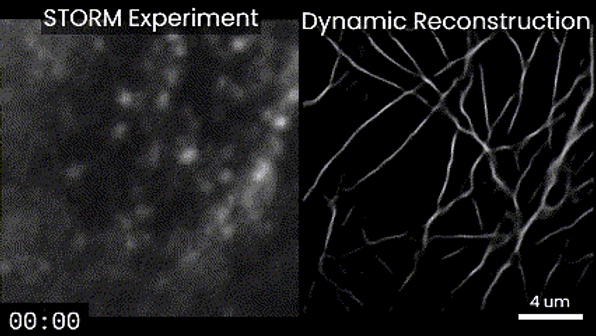
DBlink: Dynamic localization microscopy in super spatiotemporal resolution via deep learning
Single molecule localization microscopy (SMLM) has revolutionized biological imaging, improving the spatial resolution of traditional microscopes by an order of magnitude. However, SMLM techniques depend on accumulation of many localizations over thousands of recorded frames to yield a single super-resolved image, which is time consuming. Hence, the capability of SMLM to observe dynamics has always been limited. Typically, a few minutes of data acquisition are needed to reconstruct a single super-resolved frame. In this work, we present DBlink, a novel deep-learning-based algorithm for super spatiotemporal resolution reconstruction from SMLM data. The input to DBlink is a recorded video of single molecule localization microscopy data and the output is a super spatiotemporal resolution video reconstruction. We use bi-directional long short term memory (LSTM) network architecture, designed for capturing long term dependencies between different input frames. We demonstrate DBlink performance on simulated data of random filaments and mitochondria-like structures, on experimental SMLM data in controlled motion conditions, and finally on live cell dynamic SMLM. Our neural network based spatiotemporal interpolation method constitutes a significant advance in super-resolution imaging of dynamic processes in live cells.
A. Saguy, O. Alalouf, N. Opatovski, S. Jang, M. Heilemann and Y. Shechtman. "DBlink: Dynamic localization microscopy in super spatiotemporal resolution via deep learning". Nature Methods (2023)
This microtubule does not exist: Super-resolution microscopy image generation by a diffusion model
Generative models, such as diffusion models, have made significant advancements in recent years, enabling the synthesis of high-quality realistic data across various domains. Here, we explore the adaptation of a diffusion model to super-resolution microscopy images and train it on images from a publicly available database. We show that the generated images resemble experimental images, and that the generated images do not copy existing images from the training set. Additionally, we compare the performance of a deep-learning-based deconvolution method when trained on our generated data versus training on mathematical model based data and show superior reconstruction quality in means of spatial resolution. These findings demonstrate the potential contribution of generative diffusion models for microscopy tasks and pave the way for their future application in this field.
A. Saguy, T. Nahimov, M. Lehrman, O. Alalouf, Y. Shechtman. "This microtubule does not exist: Super-resolution microscopy image generation by a diffusion model". bioRxiv 2023.07.06.548004 (2023)
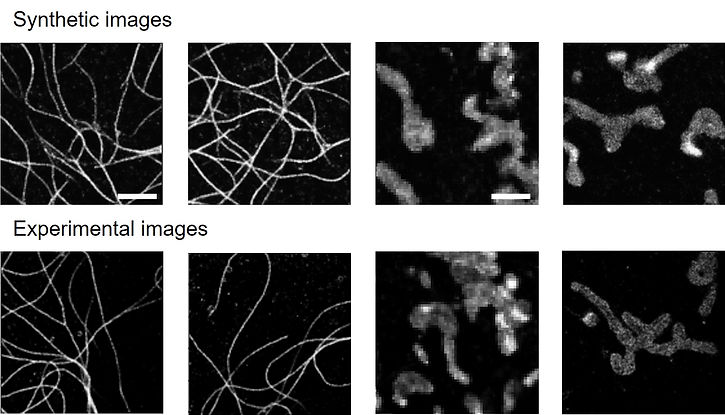
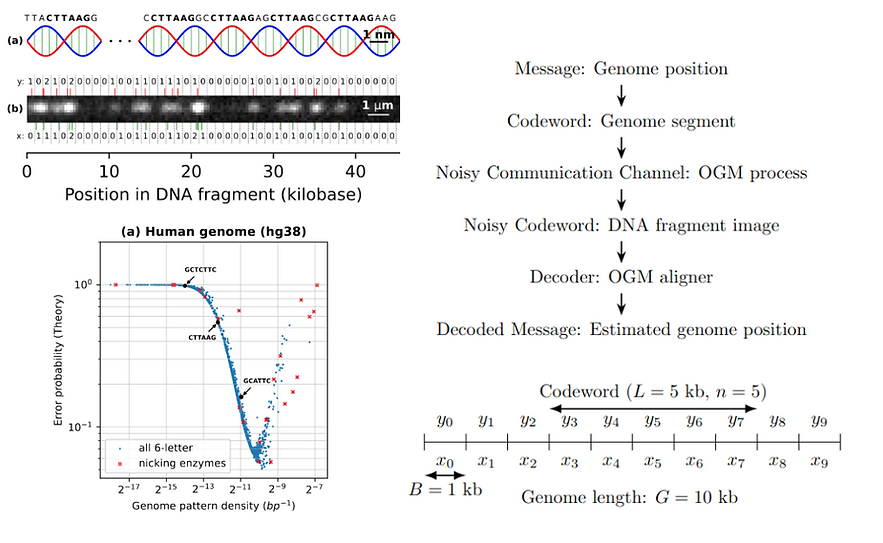
Design of optimal patterns for optical genome mapping via information theory
Optical genome mapping (OGM) is a technique that extracts partial genomic information from optically imaged and linearized DNA fragments containing fluorescently labeled short sequence patterns. This information can be used for various genomic analyses and applications, such as the detection of structural variations and copy-number variations, epigenomic profiling, and microbial species identification. Currently, the choice of labeled patterns is based on the available bio-chemical methods, and is not necessarily optimized for the application. In this work, we develop a model of OGM based on information theory, which enables the design of optimal labeling patterns for specific applications and target organism genomes. We validated the model through experimental OGM on human DNA and simulations on bacterial DNA. Our model predicts up to 10-fold improved accuracy by optimal choice of labeling patterns, which may guide future development of OGM bio-chemical labeling methods and significantly improve its accuracy and yield for applications such as epigenomic profiling and cultivation-free pathogen identification in clinical samples.
Y. Nogin, D. Bar-Lev, D. Hanania, T. D. Zur, Y. Ebenstein, E. Yaakobi, N. Wienberger, Y. Shechtman. "Design of optimal patterns for optical genome mapping via information theory". Bioinformatics 39, 10 (2023)
Learning Optimal Multicolor PSF Design for 3D Pairwise Distance Estimation
In computational microscopy, neural networks have been employed together with point spread function (PSF) engineering for various imaging challenges, specifically for localization microscopy. This combination enables “end-to-end” design of the optical system’s hardware and software, which is learned simultaneously, optimizing both the image acquisition and reconstruction together. In this work, we employ such a strategy for the task of direct measurement of the 3D distance between two emitters, labeled with differently colored fluorescent labels, in a single shot, on a single optical channel; utilizing the fact that only the distance between the two spots is of interest, rather than their absolute positions. Specifically, we use end-to-end learning to design an optimal wavelength-dependent phase mask that yields an image that is most informative with regards to the 3D distance between the two spots, followed by an analyzing net to decode this distance. We demonstrate our approach experimentally by distance measurement between pairs of fluorescent beads, as well as between 2 fluorescently tagged DNA loci in yeast cells. Our results represent an appealing demonstration of the usefulness of neural nets in task-specific microscopy design and in optical system optimization in general.
O. Goldenberg, B. Ferdman, E. Nehme, Y. S. Ezra, Y. Shechtman. "Learning Optimal Multicolor PSF Design for 3D Pairwise Distance Estimation". Intelligent Computing, Vol 2022, 0004 (2022)

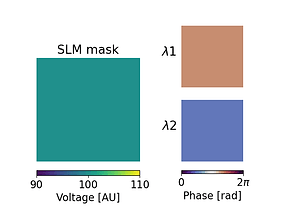


DeepOM: Single molecule optical genome mapping via deep learning
Efficient tapping into genomic information from a single microscopic image of an intact DNA molecule fragment is an outstanding challenge and its solution will open new frontiers in molecular diagnostics. Here, a new computational method for optical genome mapping utilizing Deep Learning is presented, termed DeepOM. Utilization of a Convolutional Neural Network (CNN), trained on simulated images of labeled DNA molecules, improves the success rate in alignment of DNA images to genomic references. The method is evaluated on acquired images of human DNA molecules stretched in nano-channels. The accuracy of the method is benchmarked against state-of-the-art commercial software Bionano Solve. The results show a significant advantage in alignment success rate for molecules shorter than 50 kb. DeepOM improves yield, sensitivity and throughput of optical genome mapping experiments in applications of human genomics and microbiology.
Y. Nogin, T. D. Zur, S. Margalit, I. Barzilai, O. Alalouf, Y. Ebenstein, Y. Shechtman "DeepOM: Single-molecule optical genome mapping via deep learning". Bioinformatics (2023)
Monocular kilometer-scale passive ranging by point-spread function engineering
We present monocular long-range, telescope-based passive ranging, realized by integration of point-spread-function engineering into a telescope, extending the scale of PSF engineering-based ranging to distances where it has never been tested before.
Imaging-based distance estimation is a difficult challenge, both in the microscopic and the macroscopic realms. Existing long-range distance estimation technologies mostly rely on active emission of signal, which as a subsystem, constitutes a significant portion of the complexity, size and cost of the active-ranging apparatus. Despite the appeal of alleviating the requirement for signal-emission, passive distance estimation methods are essentially nonexistent for ranges greater than a few hundreds of meters, which is the main motivation for this research. We provide experimental demonstrations of the optical system in a variety of challenging imaging scenarios, including adversarial weather conditions, dynamic targets and scenes of diversified textures, at distances extending beyond 1.7 km.
N. Opatovski, D. Xiao, G. Harari, and Y. Shechtman. "Monocular kilometer-scale passive ranging by point-spread function engineering". Optics Express, 30 (21), 37925-37937 (2022)
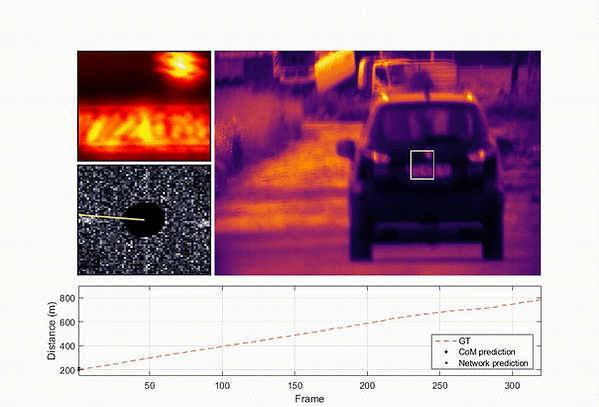
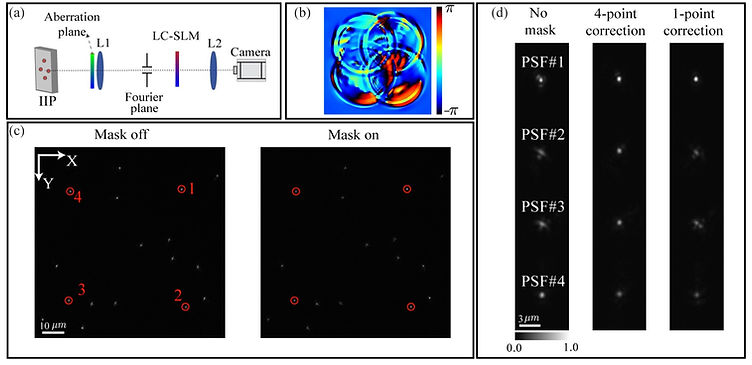
Diffractive optical system design by cascaded propagation
Modern design of complex optical systems relies heavily on computational tools. These frequently use geometrical optics as well as Fourier optics. Fourier optics is typically used for designing thin diffractive elements, placed in the system’s aperture, generating a shift-invariant Point Spread Function (PSF). A major bottleneck in applying Fourier Optics in many cases of interest, e.g. when dealing with multiple, or out-of-aperture elements, comes from numerical complexity. In this work, we propose and implement an efficient and differentiable propagation model based on the Collins integral, which enables the optimization of diffractive optical systems with unprecedented design freedom using backpropagation. We demonstrate the applicability of our method, numerically and experimentally, by engineering shift-variant PSFs via thin plate elements placed in arbitrary planes inside complex imaging systems, performing cascaded optimization of multiple planes, and designing optimal machine-vision systems by deep learning.
B. Ferdman, A. Saguy, D. Xiao, and Y. Shechtman. "Diffractive optical system design by cascaded propagation". Optics Express, 30 (15), 27509-27530 (2022)
Quantifying cell cycle dependent chromatin dynamics during interphase by live 3D tracking
The study of cell cycle progression and regulation is important to our understanding of fundamental biophysics, aging, and disease mechanisms. Local chromatin movements are generally considered to be constrained and relatively consistent during all interphase stages, although recent advances in our understanding of genome organization challenge this claim. Here we use high spatio-temporal, 4D (x, y, z + time) localization microscopy by point spread function (PSF) engineering and deep learning-based image analysis, for live imaging of mouse embryonic fibroblast (MEF 3T3) and MEF 3T3 double Lamin A Knockout (LmnaKO) cell lines, to characterize telomere diffusion during the interphase. We detected varying constraint levels imposed on the chromatin, which are prominently decreased during G0/G1. Our 4D measurements of telomere diffusion offer an effective method to investigate chromatin dynamics and reveal cell-cycle-dependent motion constraints, which may be caused by various cellular processes.
For more details:
T. Naor, Y. Nogin, E. Nehme, B. Ferdman, L. E. Weiss, O. Alalouf and Y. Shechtman. "Quantifying cell cycle dependent chromatin dynamics during interphase by live 3D tracking". iScience, 104197 (2022).
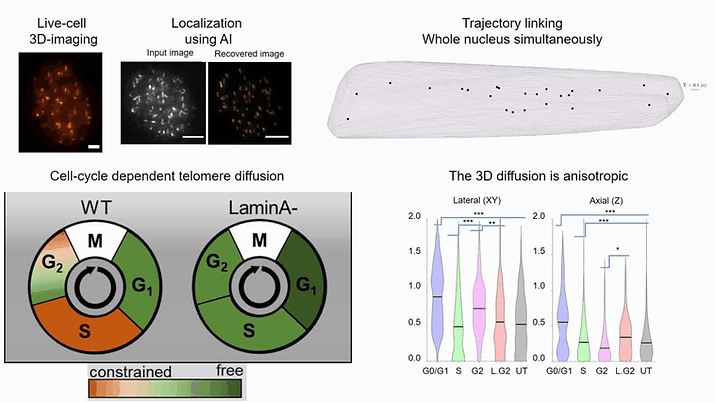

Deep-ROCS: from speckle patterns to superior-resolved images by deep learning in rotating coherent scattering microscopy
Rotating coherent scattering (ROCS) microscopy is a label-free imaging technique that overcomes the optical diffraction limit by adding up the scattered laser light from a sample obliquely illuminated from different angles. Although ROCS imaging achieves 150 nm spatial and 10 ms temporal resolution, simply summing different speckle patterns may cause loss of sample information. In this paper we present Deep-ROCS, a neural network-based technique that generates a superior-resolved image by efficient numerical combination of a set of differently illuminated images. We show that Deep-ROCS can reconstruct super-resolved images more accurately than conventional ROCS microscopy, retrieving high-frequency information from a small number (6) of speckle images. We demonstrate the performance of Deep-ROCS experimentally on 200 nm beads and by computer simulations, where we show its potential for even more complex structures such as a filament network.
For more details:
A. Saguy, F. Jünger, A. Peleg, B. Ferdman, E. Nehme, A. Rohrbach, and Y. Shechtman, "Deep-ROCS: from speckle patterns to superior-resolved images by deep learning in rotating coherent scattering microscopy", Optics Express, 29 (15), 23877-23887 (2021)
Multicolor Single-Particle-Tracking by Multiplexed PSF Engineering
PSF engineering enables 3D tracking of single sub-diffraction emitters by encoding depth into the measured image. The variability in measured PSF shapes can be further exploited to obtain spectral information of the emitters. Such benefit is obtained by separating the emission into separate spectral channels, and allotting different PSFs to each of the channels. This enables the use of single-channel phase masks, which are fairly easy to fabricate. By multiplexing the channels, spectral information is provided by the PSF while maintaining high-resolution 3D localizations. The use of multiple PSFs enables full use of the camera sensor, facilitating a large field of view.
To the right, a schematic drawing of the optical system is added, and the analysis of a large number of diffusing fluorescent beads is shown. The beads whose trajectories are shown, marked A,B,C, are visible through different spectral channels, colored by blue, orange and red respectively. The channel is determined by the orientation of the Tetrapod PSF.
For more details:
N. Opatovski, Y. S. Ezra, L. E. Weiss, B. Ferdman, R. Orange and Y. Shechtman, "Multiplexed PSF Engineering for Three-Dimensional Multicolor Particle Tracking", Nano Letters (2021)

Learning Optimal Wavefront Shaping for Multi-channel Imaging
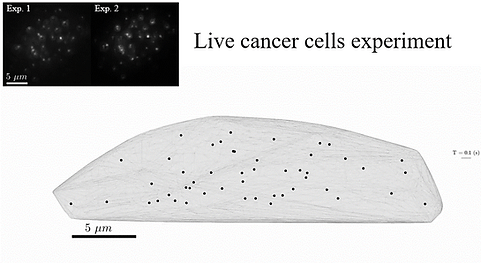
Fast acquisition of depth information is crucial for accurate 3D tracking of moving objects. Snapshot depth sensing can be achieved by wavefront coding, in which the point-spread function (PSF) is engineered to vary distinctively with scene depth by altering the detection optics. In low-light applications, such as 3D localization microscopy, the prevailing approach is to condense signal photons into a single imaging channel with phase-only wavefront modulation to achieve a high pixel-wise signal to noise ratio. Here we show that this paradigm is generally suboptimal and can be significantly improved upon by employing multi-channel wavefront coding, even in low-light applications. We demonstrate our multi-channel optimization scheme on 3D localization microscopy in densely labelled live cells where detectability is limited by overlap of modulated PSFs. At extreme densities, we show that a split-signal system, with end-to-end learned phase masks, doubles the detection rate and reaches improved precision compared to the current state-of-the-art, single-channel design. We implement our method using a bifurcated optical system, experimentally validating our approach by snapshot volumetric imaging and 3D tracking of fluorescently labelled subcellular elements in dense environments.
For more details:
E. Nehme*, B. Ferdman*, L. E. Weiss; T. Naor; D. Freedman; T. Michaeli; Y. Shechtman. "Learning optimal wavefront shaping for multi-channel imaging", in IEEE Transactions on Pattern Analysis and Machine Intelligence, doi: 10.1109/TPAMI.2021.3076873 (2021). (*equal contribution)
3D Printable Diffractive Optical Elements by Liquid Immersion
Diffractive optical elements (DOEs) are used to shape the wavefront of incident light. This can be used to generate practically any pattern of interest, albeit with varying efficiency. A fundamental challenge associated with DOEs comes from the nanoscale-precision requirements for their fabrication. Here we demonstrate a method to controllably scale up the relevant feature dimensions of a device from tens-of-nanometers to tens-of-microns by immersing the DOEs in a near-index-matched solution. This makes it possible to utilize modern 3D-printing technologies for fabrication, thereby significantly simplifying the production of DOEs and decreasing costs by orders of magnitude, without hindering performance. We demonstrate the tunability of our design for varying experimental conditions, and the suitability of this approach to ultrasensitive applications by localizing the 3D positions of single molecules in cells using our microscale fabricated optical element to modify the point-spread-function (PSF) of a microscope.
For more details:
R. Orange-Kedem, E. Nehme, L. E. Weiss, B. Ferdman, O. Alalouf, N. Opatovski and Y. Shechtman, "3D printable diffractive optical elements by liquid immersion", Nature Communications 12, 3067 (2021)
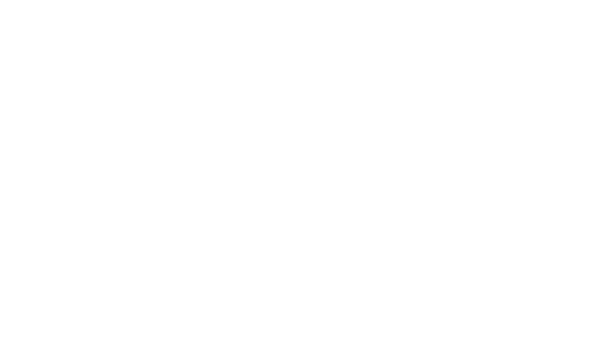
Automated Analysis of Fluorescence Kinetics in Single-Molecule Localization Microscopy Data Reveals Protein Stoichiometry
Understanding the function of protein complexes requires information on their molecular organization, specifically, their oligomerization level. Optical super-resolution microscopy can localize single protein complexes in cells with high precision, however, the quantification of their oligomerization level, remains a challenge. Here, we present a Quantitative Algorithm for Fluorescent Kinetics Analysis (QAFKA), that serves as a fully automated workflow for quantitative analysis of single-molecule localization microscopy (SMLM) data by extracting fluorophore “blinking” events. QAFKA includes an automated localization algorithm, the extraction of emission features per localization cluster, and a deep neural network-based estimator that reports the ratios of cluster types within the population. We demonstrate molecular quantification of protein monomers and dimers on simulated and experimental SMLM data. We further demonstrate that QAFKA accurately reports quantitative information on the monomer/dimer equilibrium of membrane receptors in single immobilized cells, opening the door to single-cell single-protein analysis.
Figure caption: Oligomerization state of HGF-stimulated and unstimulated MET-mEos4b receptors in a stable HEK293T cell line. (a) Temporal sum over time of a PALM experiment of a single HEK293T cell (marked with white dashed line) that expresses MET-mEos4b (scale bar = 2.5 um). The oligomerization state of MET-mEos4b was analyzed with QAFKA in resting and HGF-stimulated cells. (b) Cumulative density function of the number of blinking events without ligand stimulation and in presence of 1 nM HGF. (c) Estimation of monomer and dimer fractions with QAFKA in absence and presence of 1 nM HGF.
For more details:
A. Saguy, T. N. Baldering, L. E. Weiss, E. Nehme, C. Karathanasis, M. S. Dietz, M. Heilemann, and Y. Shechtman, "Automated Analysis of Fluorescence Kinetics in Single-Molecule Localization Microscopy Data Reveals Protein Stoichiometry", The Journal of Physical Chemistry B Article ASAP DOI: 10.1021/acs.jpcb.1c01130 (2021)

Microscopic scan-free surface profiling over extended axial ranges by point-spread-function engineering
The shape of a surface, i.e. its topography, influences many functional properties of a material; hence, characterization is critical in a wide variety of applications. Two notable challenges are profiling temporally changing structures, which requires high-speed acquisition, and capturing geometries with large axial steps. Here we leverage point-spread-function (PSF) engineering for scan-free, dynamic, micro-surface profiling. The presented method is robust to axial steps and acquires full fields of view at camera-limited framerates. We present two approaches for surface profiling implementation:
-
Fluorescence-based: using fluorescent emitters scattered on a surface of a 3D dynamic object (inflatable membrane in this example).
-
Label-free: using pattern of illumination spots projected onto a reflective sample (tilting mirror in this example).
Both implementations demonstrate the applicability to a variety of sample geometries and surface types.
For more details:
R. Gordon-Soffer, L. E. Weiss, R. Eshel, B. Ferdman, E. Nehme, M. Bercovici, and Y. Shechtman, "Microscopic scan-free surface profiling over extended axial ranges by point-spread-function engineering", Science Advances 6, 44 (2020).
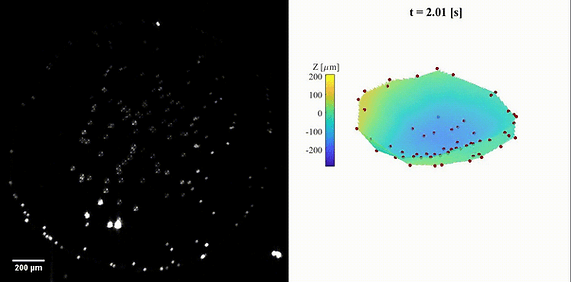
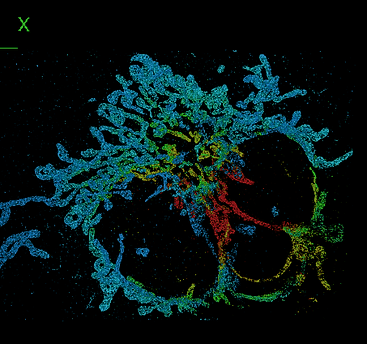
DeepSTORM3D: dense 3D localization microscopy and PSF design by deep learning
Localization microscopy is an imaging technique in which the positions of individual point emitters (e.g. fluorescent molecules) are precisely determined from their images. Localization in 3D can be performed by modifying the image that a point-source creates on the camera, namely, the point-spread function (PSF). The PSF is engineered to vary distinctively with emitter depth, using additional optical elements. However, localizing multiple adjacent emitters in 3D poses a significant algorithmic challenge, due to the lateral overlap of their PSFs. In our lastest work, DeepSTORM3D, we presented two different applications of CNNs in dense 3D localization microscopy:
-
Learning an efficient 3D localization CNN for a given PSF entirely in silico (Tetrapod in this example)
-
Learning an optimized PSF for high density localization via end-to-end optimization
For more details:
Nehme, E., Freedman, D., Gordon, R. et al. "DeepSTORM3D: dense 3D localization microscopy and PSF design by deep learning". Nature Methods (2020).
Three-dimensional localization microscopy in live flowing cells
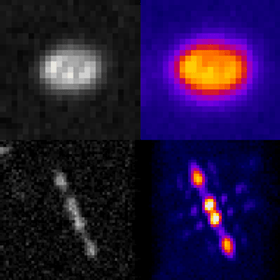
Here, we have demonstrated that by merging two technologies: point-spread-function engineering and imaging flow cytometry, we can attain excellent spatial detail with extremely high sample throughput! Essential to our approach is calibrating the imaging system. This is accomplished with a novel method that analyzes the statistical distributions of tiny fluorescent beads that are imaged alongside cells suspended in media. This is represented in the attached graphic, where the images of randomly positioned objects on the left are analyzed collectively to produce the shape-to-depth calibration on the right. This calibration is then applied to the images of fluorescently labeled positions within cells.
For more details:
L. E. Weiss, Y. Shalev Ezra, S. Goldberg, B. Ferdman, O. Adir, A. Schroeder, O. Alalouf, Y. Shechtman. "Three-dimensional localization microscopy in live flowing cells" Nature nanotechnology (2020).
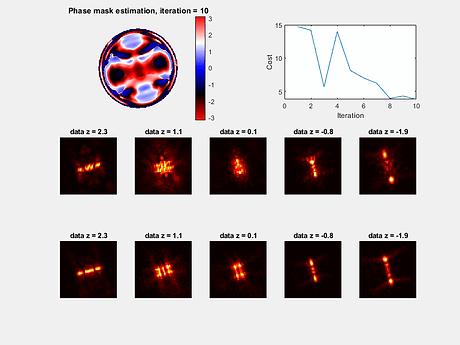
VIPR: Vectorial Implementation of Phase Retrieval
With work previously done by a former lab member, we have developed a powerful tool for use in the identification and characterization of the processes in our model system. A major advantage of this development is its improved sensitivity, which allows it to detect subtle dynamic property changes in response to our experimentation.
For more details:
B. Ferdman, E. Nehme, L. E. Weiss, R. Orange, O. Alalouf, Y. Shechtman "VIPR: Vectorial Implementation of Phase Retrieval for fast and accurate microscopic pixel-wise pupil estimation" bioRxiv (2020)
Deep learning for diffusion characterization
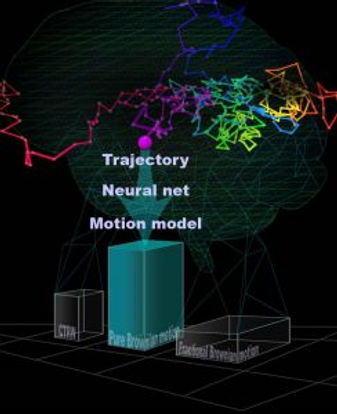
We implement a neural network to classify single-particle trajectories by diffusion type: Brownian motion, fractional Brownian motion (FBM) and Continuous Time Random Walk (CTRW). Furthermore, we demonstrate the applicability of our network architecture for estimating the Hurst exponent for FBM and the diffusion coefficient for Brownian motion on both simulated and experimental data. The networks achieve greater accuracy than MSD analysis on simulated trajectories while requiring as few as 25 steps. On experimental data, both net and MSD analysis converge to similar values, with the net requiring only half the number of trajectories required for MSD to achieve the same confidence interval.
For more details:
N. Granik, L. E. Weiss, E. Nehme, M. Levin, M. Chein, E. Perlson, Y. Roichman, Y. Shechtman "Single particle diffusion characterization by deep learning", Biophysical Journal 117, 2,185-192 (2019).
Ultrasensitive refractometry

We present a refractometry approach in which the fluorophores are preattached to the bottom surface of a microfluidic channel, enabling highly-sensitive determination of the Refractive Index using tiny amounts of liquid by detecting the Supercritical Angle Fluorescence (SAF) effect at the conjugate back focal plane of a high NA -obejective.
The SAF effect (presented above) is the propagation of evanescent waves in the higher refractive index immersion medium, which captures the change in the transfer coefficients, observed as a strong transition ring.
We demonstrate the relevance of our system for monitoring changes in biological systems. As a model system, we show that we can detect single bacteria (Escherichia coli) and measure population growth.
For more details:
B. Ferdman*, L.E. Weiss*, O. Alalouf, Y. Haimovich, Y. Shechtman "Ultrasensitive refractometry via supercritical angle fluorescence", ACS Nano, 12, 11892-11898 (2018). (*Equal Contribution)
*** See also ACS Nano perspective (12/2018)
Deep-learning for super-resolution localization microscopy
Deep-learning for multicolor localization microscopy
Deep learning has been shown to be an effective tool for image classification. Here we demonstrate that capability extends to distinguishing the colors of single emitters from grayscale images as well. This was done by training a convolutional neural network (CNN) on a library of images containing up to four types of quantum dots with different emission wavelengths embedded in a polymer matrix, then evaluating the net with new images. Surprisingly, we found that the same approach was applicable to the much more challenging problem of classifying moving emitters as well, where the chromatic-dependent subtleties in the point-spread function (PSF) are distorted by motion blur. The performance of the neural net in these two applications show that such an approach be used to simplify the design of multicolor microscopes by replacing hardware components with downstream software analysis.
In a second application of neural nets, we have shown how a phase-modulating element, which can be used to control the shape of the PSF, can be designed in parallel with net training in order to optimize the ability of the net to distinguish the position and color of the object. This approach produces novel phase masks that increase the net’s ability to categorize emitters while maintaining other desirable properties, namely, the localizability of emitters. This approach for mask optimization solves a longstanding problem in PSF-engineering: how a phase mask can be optimally designed to encode for any parameter of interest.
For more details:
E. Hershko, L.E. Weiss, T. Michaeli, Y. Shechtman, “Multicolor localization microscopy and point-spread-function engineering by deep learning“, Optics Express 27, 5, 6158-6183 (2019)
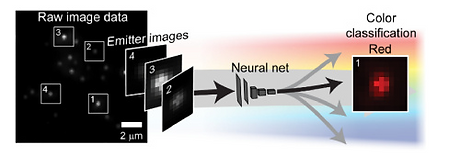

Deep-STORM: Super resolution single molecule microscopy by deep learning
In localization microscopy, regions with a high density of overlapping emitters pose an algorithmic challenge. Various algorithms have been developed to handle overlapping PSFs, all of which suffer from two fundamental drawbacks: data-processing time and sample-dependent paramter tuning.
Recently we demonstrated a precise, fast, parameter-free, super-resolution image reconstruction by harnessing Deep-Learning. By exploiting the inherent additional information in blinking molecules, our method, dubbed Deep-STORM, creates a super-resolved image from the raw data directly. Deep-STORM is general and does not rely on any prior knowledge of the structure in the sample.
For more details:
E. Nehme, L.E. Weiss, T. Michaeli, and Y. Shechtman, "Deep-STORM: Super Resolution Single Molecule Microscopy by Deep Learning", Optica 4, 458-464 (2018).
*** See also Nature Methods highlight ( May 2018)
Background: Optimal-3D and multicolor PSF engineering
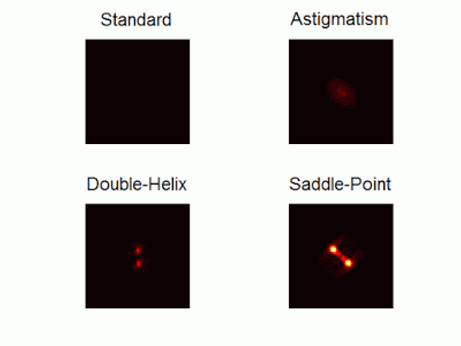
Optimal-3D and multicolor PSF engineering
How, and to what precision, can one determine the 3D position of a sub-wavelength particle by observing it through a microscope? This is the problem at the heart of methods such as single-particle-tracking and localization based super-resolution microscopy (e.g. PALM, STORM). One useful way of achieving such 3D localization at nanoscale precision is to modify the point-spread-function (PSF) of the microscope so that it encodes the 3D position in its shape.We have recently asked the basic question – what is the optimal way to modify a microscope’s PSF in order to encode the 3D position (x,y,z) of a point emitter in the most efficient way? We approach this challenge by solving an optimization problem: Find a pupil-plane phase pattern that yields a PSF which is maximally sensitive to small changes in the particle’s position. Formulated mathematically, this sensitivity corresponds to the Fisher-Information of the system. The result is the saddle-point PSF (bottom right panel in figure above)
For more details:
Y. Shechtman, S.J. Sahl, A.S. Backer and W.E. Moerner, "Optimal point spread function design for 3D imaging", Physical Review Letters 113, 133902 (2014).
Extremely-large-range PSFs for 3D localization microscopy
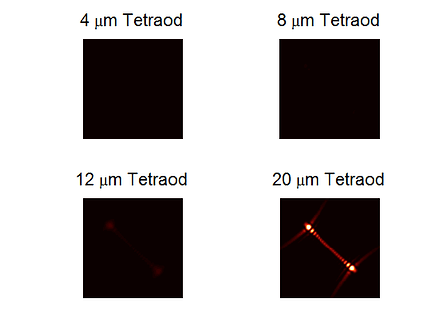
Our PSF optimization method can be used to generate PSFs with unprecedented capabilities, like an extremely large, modular axial (z) range of up to 20 um. The resulting optimal large-range PSFs belong to a family of PSFs we call the Tetrapod PSFs (see image above)
Simulation of different Tetrapod PSFs as a particle is scanned over a 20 micron axial (z) range (from -10 um to +10 um and back)
We demonstrate experimentally the applicability of these Tetrapod PSFs in micro-fluidic flow profiling over a 20um z range, and in tracking under noisy biological conditions.
For more details:
Y. Shechtman, L.E. Weiss, A.S. Backer, S.J. Sahl and W.E. Moerner, "Precise 3D scan-free multiple-particle tracking over large axial ranges with Tetrapod point spread functions", Nano Letters,DOI:10.1021/acs.nanolett.5b01396 (2015).
Multicolor 3D PSFs
Often in fluorescent microscopy one is interested in observing several different types of fluorescently labeled objects. Commonly, this is done by labeling different objects with different colors. How can you distinguish between different colors using a highly sensitive grayscale detector (e.g. EMCCD)?
One approach is to separate the emission light into different color channels with dichroic elements. Alternatively, is possible to switch between emission filters and image sequentially. But how do you simultaneously image multiple colors on a single multiple channel with no additional elements (other than a 4F system with a phase mask)? The answer is multicolor PSF engineering.
By exploiting the spectral response of the phase modulating element, it is possible to design masks that create different phase delays for different colors, and therefore enable simultaneous multicolor 3D tracking or multicolor super-resolution imaging.
For more details:
Y. Shechtman, L.E. Weiss, A.S. Backer, M.Y. Lee and W.E. Moerner, "Multicolor localization microscopy by point-spread-function engineering“,Nature Photonics 10, (2016).
*** See also Nature Methods highlight ( September 2016)
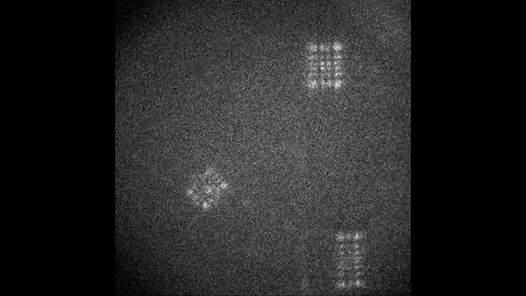